Background
The overarching goal of this project is to achieve a technological breakthrough in (multi-agent) cooperative lifelong learning theory and practice, so as to accomplish adversary-resilient, fast-adaptable, and communication/computation-efficient multimodal information fusion for distributed sensing systems in dynamic environments.
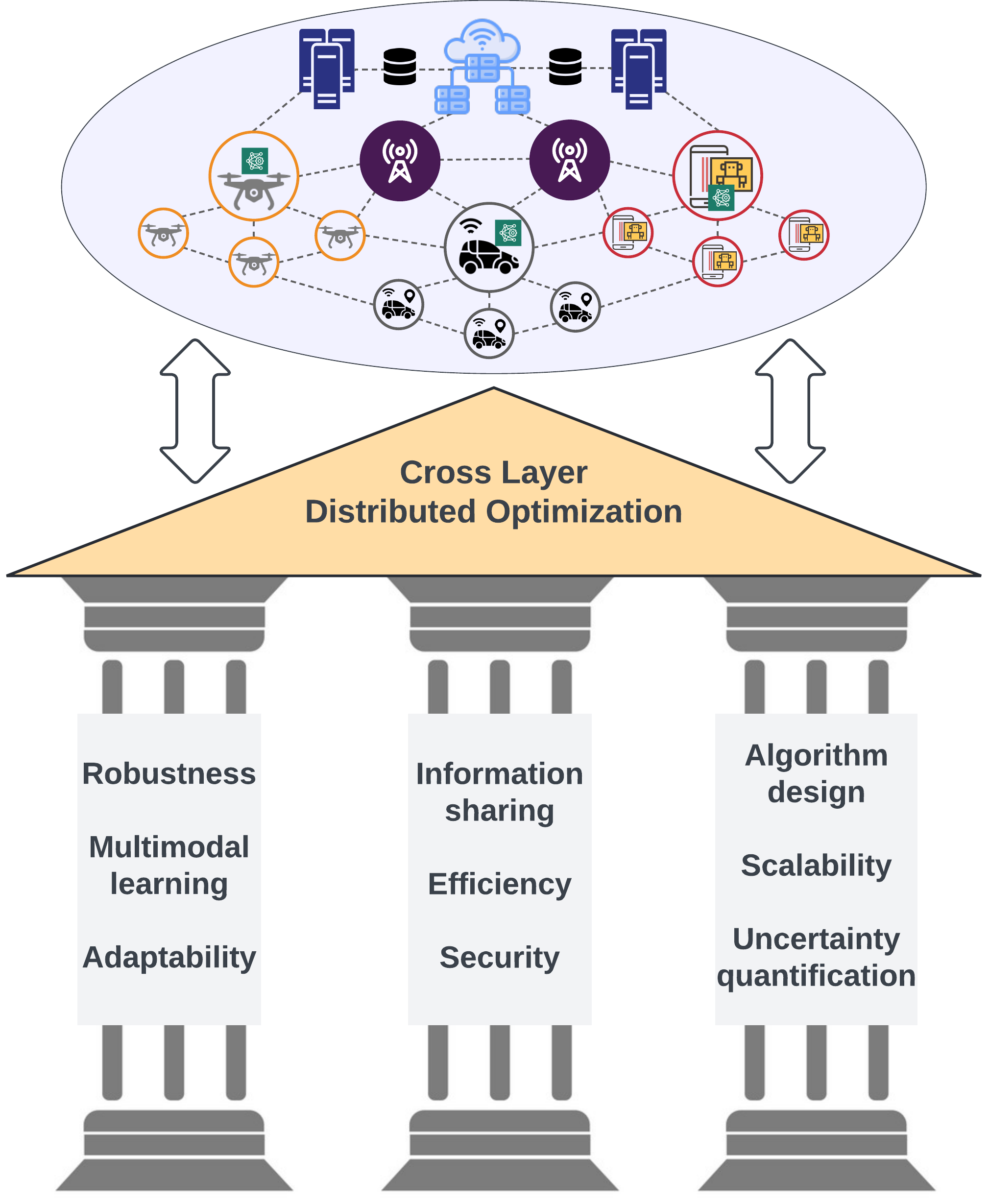
Cross-Layer Distributed Optimization
We aim to develop cross-layer distributed optimization (CLDO), a new framework where networked agents process data to solve a multi-level optimization problem, addressing needs like prediction accuracy, robustness, data-model efficiency, and quick environmental adaptation. This approach efficiently utilizes shared experiences, enabling fast adaptation in dynamic, multifaceted environments.
This project comprises three main thrusts:
- Thrust I (Intra-agent lifelong learning): We focus on achieving lifelong, robustness-aware learning from time-evolving multi-modal data.
- Thrust II (Data-model efficiency): We aim to achieve data-model efficiency for lossless learning across multiple agents.
- Thrust III (Scalable cross-layer optimization): We aim to enhance the optimization foundation of CLDO to make it more practical and scalable.
Lifelong Learning
The continuous and adaptive ability of a model to learn from new data over time, incorporating new knowledge while retaining previously learned information.
Robustness
The resilience of a model to make accurate predictions despite the presence of intentionally crafted inputs designed to cause errors or misjudgments.
Data-Model Efficiency
The optimized balance between the complexity of a machine learning model and the amount of data required, ensuring effective learning and generalization with minimal computational resources and data.
Distributed Learning
Networked agents collectively process the gathered data to solve a multi-level optimization problem formulated to simultaneously fulfill various learning requirements.
Research Team
PIs
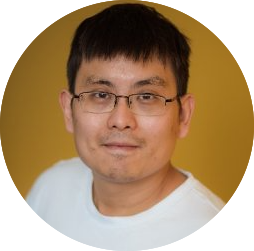
Dr. Sijia Liu
PI, Assisitant Professor, MSUDr. Sijia Liu is an Assistant Professor at the CSE department of Michigan State University, and an Affiliated Professor at the MIT-IBM Watson AI Lab, IBM Research. His research spans the areas of machine learning, optimization, computer vision, signal processing and computational biology, with a focus on scalable and trustworthy AI. He received the Best Paper Runner-Up Award at UAI 2022, and the Best Student Paper Award at ICASSP 2017.
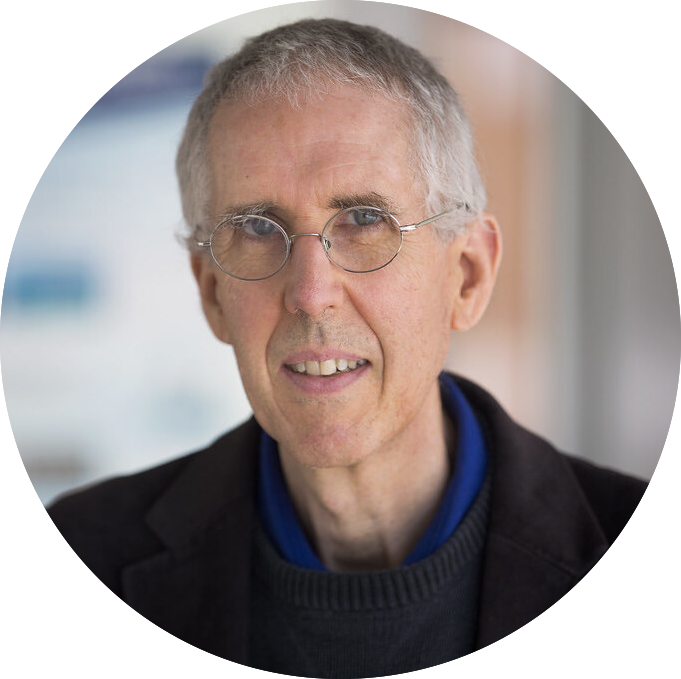
Dr. Alfred O. Hero
CO-PI, Distinguished Professor, UMDr. Alfred Hero is the John H. Holland Distinguished University Professor of EECS and the R. Jamison and Betty Williams Professor of Engineering at the University of Michigan, Ann Arbor. His research is on data science and developing theory and algorithms for multimodality data collection, fusion, analysis and visualization that use statistical machine learning and distributed optimization. He is a Fellow of IEEE and received the Fourier Award in Signal Processing from the IEEE.
Postdoc Research Fellow
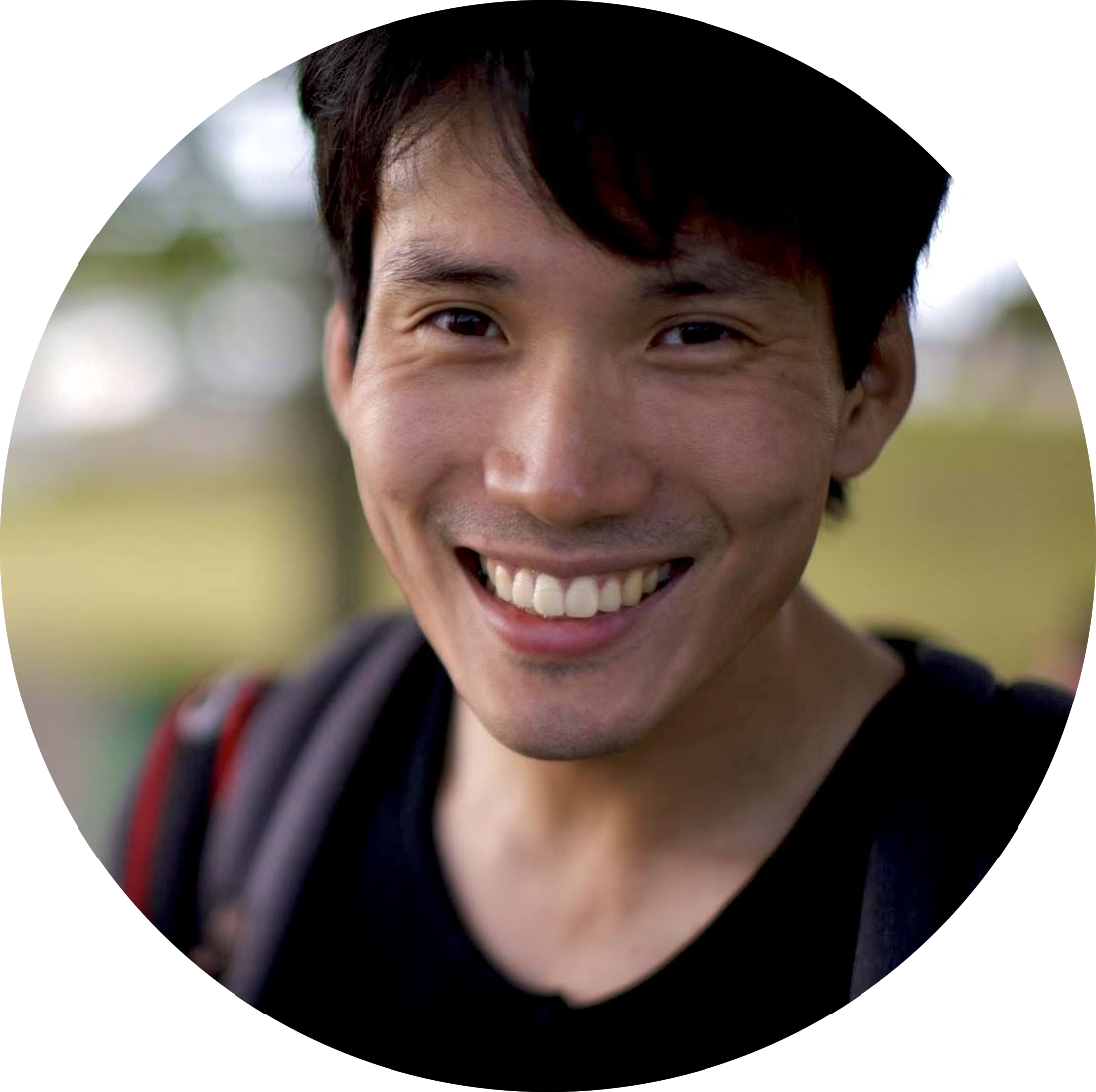
Dr. Dogyoon Song
Postdoc, UMGraduate Research Assistants
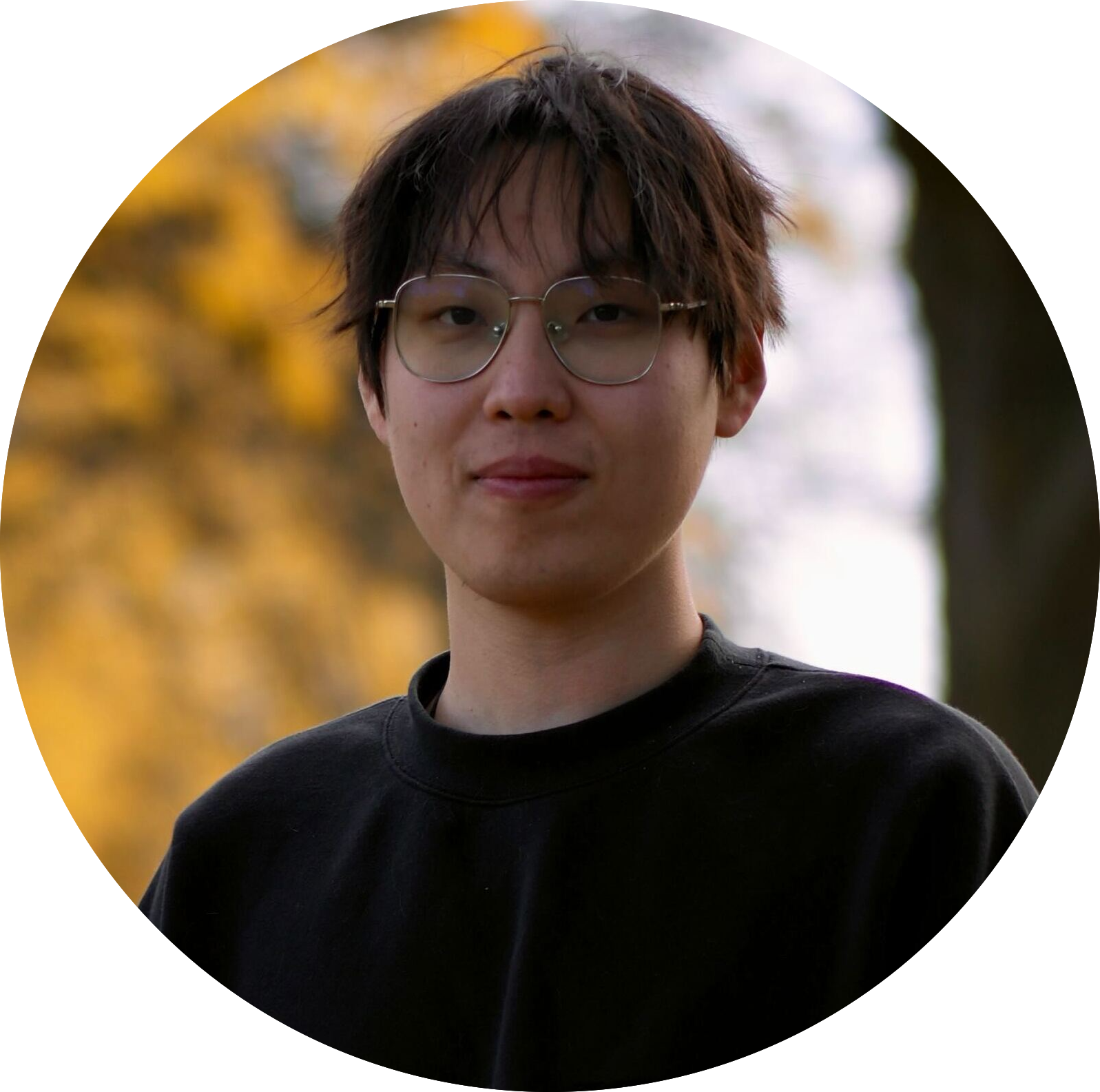
Jinghan Jia
Graduate Student, MSU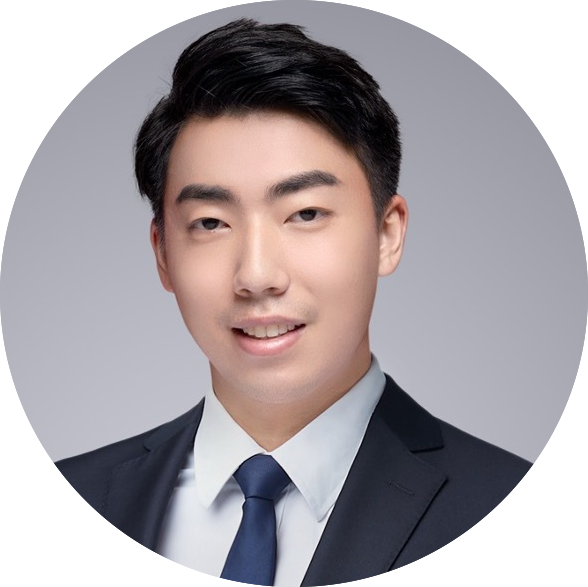
Yihua Zhang
Graduate Student, MSU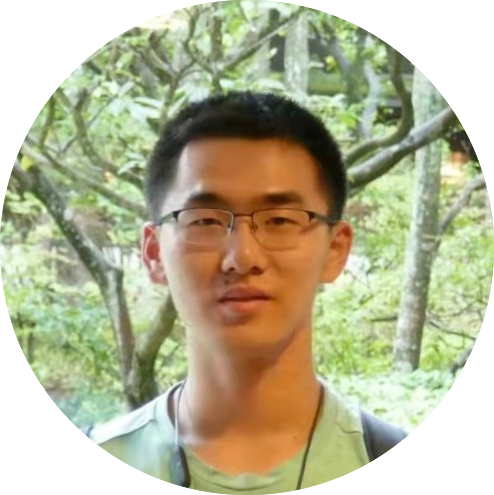
Zeyu Sun
Graduate Student, UM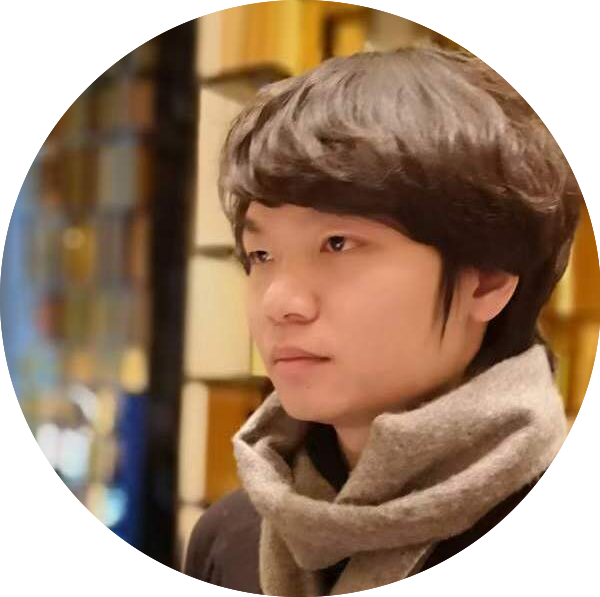
Jiancheng Liu
Graduate Student, MSU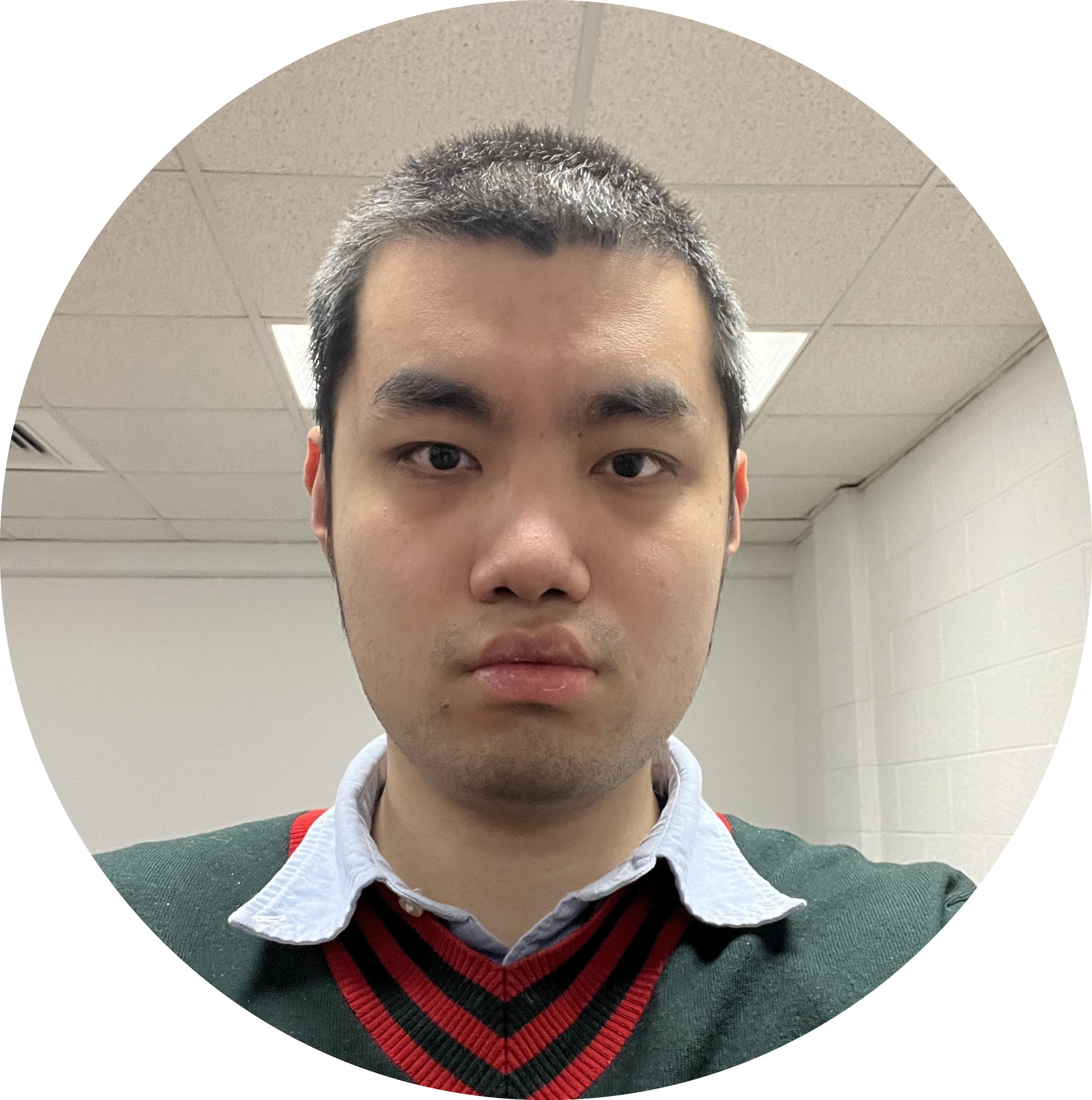